ORiGINAL RESEARCH
ENCEVIS Automated Seizure Detection Performance: Influence of Seizure Characteristics
ABSTRACT
Background: Long-term video-EEG monitoring (LTM) in dedicated epilepsy units increases the chances of capturing epileptic seizures. It is thus essential for diagnosing epilepsy, seizure classification, and patient selection for epilepsy surgery. LTM recordings generate vast data that require EEG experts to assess visually, which is time-consuming and expensive. Several automatic seizure detection systems based on scalp EEG are available. One such system is the ENCEVIS. Our study approaches how the ENCEVIS software could be used in an EMU setting. We covered a wide range of epilepsy syndromes, providing excellent insight into the sensitivity of ENCEVIS across various forms of epilepsy commonly seen in clinical practice.
Objectives: To assess seizure characteristics that influence the ENCEVIS's performance, we compared its performance to conventional EEG review by clinical physiologists according to seizure onset localization, duration, electrographic changes, and semiology.
Methods: We prospectively included 266 anonymous video-EEG recordings (>4h) from 263 individuals ages 4-67 in the study. The final database contained 112 seizures lasting more than 5 seconds from 43 ictal recordings. Recordings with non-epileptic paroxysmal events were excluded.
Two independent EEG experts visually analyzed and assessed ENCEVIS. They defined true positive, false negative, and false positive seizure detections.
Results: Seizure duration (<10 sec 6.3%, >60 sec. 63.9% (p<0.05) influenced ENCEVIS sensitivity.
GTCS and FB-TCS were detected with 100% sensitivity. Focal seizures with impaired awareness also were detected as statistically reliable at 69.5% (P<005). Sensitivities for focal aware seizure and generalized tonic seizures were 26.7% and 23.1%, respectively.
Conclusions: ENCEVIS software does not perform as well in real-life scenarios as clinical physiologists do. The algorithm is most sensitive to longer-duration focal seizures with impaired awareness, GTCS, and FB-TCS seizures. Sensitivity is the lowest for shorter generalized tonic and focal aware seizures. This assessment may give future users helpful insight into this software's strengths and weaknesses and help further improve the algorithm.
Keywords: Automatic seizure detection; epilepsy; long-term video EEG monitoring; seizure.
DOI: 10.52340/GBMN.2024.01.01.82
BACKGROUND
Seizure recording using video-EEG is essential for diagnosing epilepsy, differentiating epilepsy from other conditions, classifying seizures, and selecting patients for epilepsy surgery.1,2 Long-term video-EEG monitoring (LTM) in dedicated epilepsy units increases the chances of capturing epileptic seizures and accompanying EEG epileptiform activity.3 LTM recordings generate vast data that require EEG experts to assess visually. This process is both time-consuming and expensive.
Several studies have demonstrated that algorithms for automatic seizure detection (ASD) and automatic detection of interictal epileptiform patterns have high sensitivity and specificity; however, those studies contain only a small number of patients or cannot be replicated.4-6
Several automatic seizure detection systems based on scalp EEG are available. One such system is the ENCEVIS system, developed by the Austrian Institute of Technology (AIT).7 Promising results were obtained from studies conducted in the EMU. According to a study by Johannes Koren et al., commercially available seizure-detection software packages showed similar and reasonable sensitivities when using the same data set. In this study, ENCEVIS 1.7 demonstrated slightly lower sensitivity but the highest specificity.8,9,13
To assess seizure characteristics that influence the performance of the ENCEVIS 1.7, we compared its performance to conventional EEG review by clinical physiologists according to seizure onset localization, duration, electrographic changes, and semiology.
METHODS
We prospectively included 266 anonymous video-EEG recordings (>4h) from 263 individuals, ages 4-67 years, from 2018 to 2022 recorded at S. Khechinashvili University Hospital. In the final analysis, we included 43 recordings containing at least one epileptic seizure. Recordings of patients with non-epileptic paroxysmal events were not included. Additionally, only electroclinical seizures lasting more than 5 seconds were considered for the final analysis.
​
The EEG was recorded using the Micromed EEG system (System PLUS Evolution 1.04.215, Micromed S.p.A., Veneto, Italy). Polygraphic electrodes were also utilized, including EOG, chin EMG, and ECG. It is important to note that the seizure detection system ENCEVIS was actively running online alongside the video-EEG monitoring (VEM) process.
​
We studied the commercially available ENCEVIS 1.7 software package. ENCEVIS employs a multimodal approach to seizure detection using EEG features with ECG.7-10 We used only the software's seizure detection features, ignoring other tools such as detecting different interictal patterns, EEG amplitude, etc. ENCEVIS 1.7. was running online with video-EEG monitoring.
​
The recordings were copied into two separate databases without any processing. Later, two independent EEG experts performed a visual analysis and assessment of ENCEVIS. The experts who analyzed the EEG data did not have access to ENCEVIS annotations. On the other hand, the experts who assessed the detection results of the seizure-detection software were blind to the video-EEG reports of visual analysis. All experts involved in the study had over ten years of experience in epileptology and were certified as clinical neurophysiologists by the Ministry of Health in Georgia.
​
EEG experts identified the onset of seizures by using the first detectable electrographic change during clinical seizures. They defined actual seizure detection as repetitive epileptiform EEG discharges of >2 Hz or a characteristic pattern showing a quasi-rhythmic spatiotemporal evolution (i.e., a gradual change in frequency, amplitude, morphology, or location).11 The experts determined markers for seizure onset and termination, extracted for each seizure in all patients. These markers were accepted as the reference standard, and annotations made by ENCEVIS 1.7 were compared to them. The durations of all ictal EEG patterns were identified. We regarded recording in which any seizure was detected as positive for epilepsy, regardless of whether all seizures in that record had been identified.
​
During the evaluation of ENCEVIS performance, a seizure detection was considered true positive (TP) when ENCEVIS detected it within 30 seconds before the seizure onset and 60 seconds after the seizure termination. False negatives (FN) - when the experts detected seizures, but ENCEVIS missed them. False positives (FPs) occur when ENCEVIS incorrectly annotates EEG as seizures. The sensitivity is calculated as the TP/(TP+FN) ratio.14 Moreover, the specificity was measured as the number of false positives per hour (FP/h).
​
We distinguished between seizures lasting less than 10 seconds and those lasting 10-60 seconds or longer than 60 seconds. We also studied algorithm performance according to seizure type, and seizure classification was determined using the latest ILAE classification.12 Finally, we estimated the false positive rate per hour.
​
The statistical analysis involved using the standard SPSS 27 software to calculate the mean values and standard deviations (mean ± SD) of the variables for the patients in the study group. The tests were performed with a 95% confidence interval.
​
One-way Analysis of Variance (ANOVA) was employed to evaluate the differences in the distribution of parametric indicators based on various criteria.
​
Furthermore, analysis of covariance was utilized to evaluate changes in seizure detection rates according to seizure duration; values were considered reliable if the statistical confidence coefficient p was less than 0.05 with a 95% confidence interval.
RESULTS
Two hundred sixty-six video EEGs longer than four hours were selected. The mean duration was 11.06 hours (4 to 48 hours), resulting in 2690 hours of analyzed data. Fifty-four recordings contained clinical events, 11 recordings were excluded (10 recordings with non-epileptic paroxysmal events and one with both epileptic and non-epileptic paroxysmal events) 43 recordings contained at least one electroclinical seizure identified by reference standards and were included in the study. These 43 recordings accounted for 112 visually documented seizures. The median seizure count was 2.6 per recording (range 1-17). Out of these, 76 seizures had a focal onset (average 2.3 per recording), and 36 had a generalized onset (average 3.4 per recording).
​
In the study population, the distribution of epilepsy based on seizure onset was as follows: 30 patients (69.7%) had Focal epilepsy, out of which 12 (27.9%) had frontal lobe epilepsy (FLE), 14 (32.55%) had temporal lobe epilepsy (TLE), and 4 (9.3%) had parietal/occipital lobe epilepsy (P/OLE). Additionally, 13 patients (30.25%) had Generalized epilepsy syndromes.
​
ENCEVIS accurate positive seizure detections per recording varied from 0% to 100%, with a mean of 71.2%. For focal epileptic seizures, the average sensitivity was 75.1% (frontal lobe onset – 73.8%, temporal lobe onset - 76.2%, and occipital/parietal lobe onset - 75%). The mean sensitivity in the generalized seizure onset subgroup was 62%.
​
ENCEVIS had a mean false positive (FP) detection rate of 6.3 per recording. The mean false negative (FN) detection rate was 1.4 per recording. The rate of false positive detections per hour varied from 0/hour to 2.2/hour, with a mean of 0.35/hour (0.35±0.50). Most false positives were non-seizure-related tachycardia, muscle artifacts, movement artifacts, or interictal activity.
​
Of the 43 recordings containing seizures, ENCEVIS recognized at least one seizure in 34 or 79.1% of the recordings containing seizures. Moreover, ENCEVIS did not make any FP or FN annotations in one of the recordings.
​
ENCEVIS performance per seizure
Reference standard visual analysis identified 112 electroclinical seizures. Out of the 112 visually detected seizures, ENCEVIS correctly marked 58 of them, resulting in a true positive (TP) detection rate of 51.8%.
​
Localization of the epileptogenic zone is indeed a crucial aspect of epilepsy diagnosis. In our analysis, we compared ENCEVIS detection sensitivity based on the localization of seizure onset (Tab.1). The sensitivity for seizures with frontal lobe onset was 53.5%. The sensitivity for seizures with parietal/occipital lobe onset was 75%, although this result is based on a small sample size of only four seizures, making it less reliable and insignificant. For seizures with temporal lobe onset, the sensitivity was 69%. The comparison of the true positive (TP) and false negative (FN) rates demonstrated that seizures of temporal lobe onset were statistically reliable with a p-value of less than 0.05 (p<0.05). That suggests ENCEVIS demonstrated more consistent and accurate performance in detecting temporal lobe seizures than seizures from extratemporal localization.
TABLE 1. Influence of seizure onset zone on the ENCEVIS per seizure sensitivity
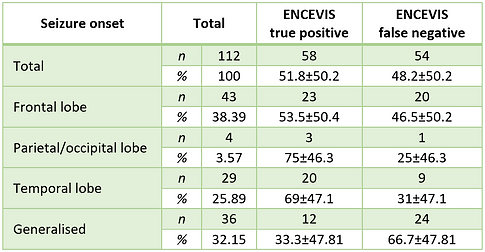
Our study revealed a positive correlation between ENCEVIS sensitivity and seizure duration. The total number of seizures analyzed was 112. For short seizures lasting below 10 seconds, the sensitivity was lowest at 6.3%; for seizures ranging from 10 to 60 seconds, the sensitivity was 55.7%; seizures longer than 60 seconds had the highest sensitivity at 63.9% (p<0.05). Thus, detection performance by ENCEVIS positively correlates with seizure duration (Tab.2).
TABLE 2. Influence of seizure duration on the ENCEVIS per seizure
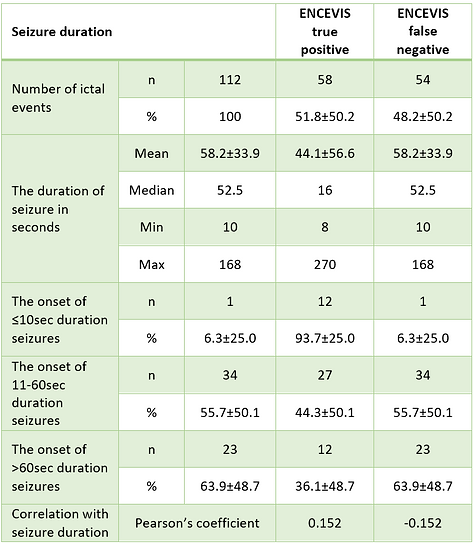
Seizure type influenced algorithm detection, statistically reliable detection was found for GTCS and FB-TCS 100% (P<0.05) and focal seizures with impaired awareness mean duration 67.07±52.34 sec 65.5% (P<0.05) (Tab.3). Seizure detection was low for subtle tonic seizures, with a mean duration of 11.81±3.3 sec., 23.1% mostly recorded from patients with learning disabilities. Missed tonic seizures had somewhat shorter duration <10 seconds, and on the EEG, ictal rhythmic patterns were not observed.
TABLE 3. Influence of seizure type on the ENCEVIS per seizure sensitivity
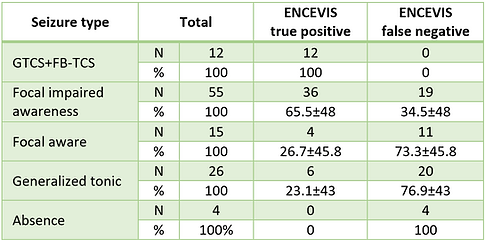
Abbreviations: FB-TCS, focal bilateral tonic-clonic seizures; GTCS, generalized tonic-clonic seizures.
DISCUSSION
In our study, we approach how the software would be used in an EMU setting by comparing the performance of the clinical physiologists with that of the software. We addressed various epilepsy syndromes, offering valuable insight into the effectiveness of ENCEVIS across common forms of epilepsy in clinical practice.
​
The ENCEVIS had an average sensitivity of 71.2% per recording, with the highest sensitivity observed for temporal lobe onset seizures (76.2%). However, the recordings from patients with generalized epilepsy had significantly lower sensitivity (62%). These findings are consistent with previously published data.8,10
​
Furthermore, our database contained different seizure types; GTCS and FB-TCS were detected with 100% sensitivity. Focal seizures with impaired awareness also were detected as statistically reliable at 69.5% (P<005). Sensitivities for focal aware seizure and generalized tonic seizures were lower, 26.7 and 23.1, respectively. This was because they usually have no or short EEG correlates. The present study and previous reports suggest that detection software does not perform as well as clinical physiologists.7-9
​
The results demonstrated that false positives occur at a rate of 0.35 per hour, consistent with previous studies.7-10 The background EEG activity may influence ENCEVIS's detection of false positives. Therefore, additional studies are needed to identify factors affecting the FP detection rate.
​
In this study, we also examined the potential of ENCEVIS as a screening tool. Our findings show that ENCEVIS correctly identified at least one seizure in 79.1% of recordings that contained seizures. This is a significant result because it suggests that ENCEVIS 1.7 can assist neurophysiologists in identifying recordings of epileptic seizures from a large dataset, thus reducing their workload. However, experts must always check and verify the algorithms' detections.
​
Our study has some limitations. It is a single-center study, and results may differ in other settings. We only focused on seizure detection. Furthermore, the online usability of these detection software packages should be investigated, as they might be beneficial for patient safety and ictal testing.
CONCLUSIONS
Although the performance of ENCEVIS software is less reliable than that of clinical physiologists, it can be used in an EMU setting if the user is aware of its limitations.
The algorithm is most sensitive to focal seizures with impaired awareness and GTCS and FB-TCS seizures and least sensitive to generalized tonic focal aware seizures.
ENCEVIS can detect at least one seizure in the ictal EEG recordings with a sensitivity of 79.1%, making it a valuable tool for automated screening and identification of such recordings. This has the potential to save time and reduce the workload of neurophysiologists.
This assessment may give future users helpful insight into this software's strengths and weaknesses and help further improve the algorithm.
AUTHOR AFFILIATION
1 Khechinashvili University Hospital (SKUH), Tbilisi, Georgia;
2 Department of Neurology, Tbilisi State Medical University, Tbilisi, Georgia.
ACKNOWLEDGEMENTS
We extend our deepest gratitude to SEIN (Stichting Epilepsie Instellingen Nederland) for its collaborative partnership and support in this study and to Al de Weerd from SEIN, for his expertise in clinical neurophysiology and relentless support in creating protocols and reviewing data. We want to thank Tilmann Kluge from the Center for Health & Bioresources (Austrian Institute of Technology GmbH, Vienna, Austria) for his relentless support in solving technical issues during the project.
REFERENCES
-
Shih JJ, Fountain NB, Herman ST, Bagic A, Lado F, Arnold S, et al. Indications and methodology for video-electroencephalographic studies in the epilepsy monitoring unit. Epilepsia2018;59:27–36. https://doi.org/10.1111/epi.13938.
-
Fountain NB, Freeman JM. EEG is an essential clinical tool: pro and con. Epilepsia2006;47:23–5. https://doi.org/10.1111/j.1528-1167.2006.00655.x.
-
Friedman DE, Hirsch LJ. How long does it take to make an accurate diagnosis in an epilepsy monitoring unit? Clin Neurophysiol2009;26:213–7. https://doi.org/10.1097/WNP.0b013e3181b2f2da.
-
Baumgartner, C., Koren, J. P., &Rothmayer, M. (2018). Automatic computer-based detection of epileptic seizures. Frontiers in Neurology, 9, 639. https://doi.org/10.3389/fneur.2018.00639
-
Da Silva Lourenço, C., Tjepkema-Cloostermans, M. C., & van Putten, M. J. A. M. (2021). Machine learning for detection of interictal epileptiform discharges. Clinical Neurophysiology, 132(7), 1433-1443. https://doi.org/10.1016/j.clinph.2021.02.403
-
Rubboli, G., Beniczky, S., Claus, S., et al. (2015). A European survey on current practices in epilepsy monitoring units and implications for patients' safety. Epilepsy &Behavior, 44, 179-184. DOI:10.1016/j.yebeh.2015.02.004
-
Fürbass, F., Ossenblok, P., Hartmann, M., Perko, H., Skupch, A. M., Lindinger, G., et al. (2015). Prospective multi-center study of an automatic online seizure detection system for epilepsy monitoring units. Clinical Neurophysiology, 126(6), 1124-1131. https://doi.org/10.1016/j.clinph.2014.09.023
-
Koren, J., Hafner, S., Feigl, M., Baumgartner, C. (2021). Systematic analysis and comparison of commercial seizure-detection software. Epilepsia, 62, 426-438. https://doi.org/10.1111/epi.16812
-
Reus, E. E. M., Visser, G. H., van Dijk, J. G., & Cox, F. M. E. (2022). Automated seizure detection in an EMU setting: Are software packages ready for implementation? Seizure, 96, 13-17. https://doi.org/10.1016/j.seizure.2022.01.009
-
Fürbass, F., Kampusch, S., Kaniusas, E., Koren, J., Pirker, S., Hopfengärtner, R., Stefan, H., Kluge, T., & Baumgartner, C. (2017). Automatic multimodal detection for long-term seizure documentation in epilepsy. Clinical Neurophysiology, 128, 1466-1472. https://doi.org/10.1016/j.clinph.2017.05.013
-
Kane N, Acharya J, Beniczky S, Caboclo L, Finnigan S, Kaplan PW, et al. A revised glossary of terms most commonly used by clinical electroencephalographers and updated proposal for the report format of the EEG findings. Revision 2017. Clin NeurophysiolPrac 2017; 2:170–85. https://doi.org/10.1016/j.cnp.2017.07.002.
-
Fisher RS, Cross JH, French JA, Higurashi N, Hirsch E, Jansen FE, et al. Operational classification of seizure types by the international league against epilepsy: position paper of the ILAE commission for classification and terminology. Epilepsia 2017; 58:522–30. https://doi.org/10.1111/epi.13670.
-
Kamitaki BK, Yum A, Lee J, Rishty S, Sivaraaman K, Esfahanizadeh A, Mani R, Wong S. Yield of conventional and automated seizure detection methods in the epilepsy monitoring unit. Seizure 2019; 69:290–5. https://doi.org/10.1016/j.seizure.2019.05.019.
-
Aleksandre Tsereteli, Natela Okujava, Nikoloz Malashkhia, Konstantine Liluashvili, Al de Weerd, The ENCEVIS algorithm in the EMU and the factors affecting its performance: Our experience, Volume 26, 2024, 100656, https://doi.org/10.1016/j.ebr.2024.100656